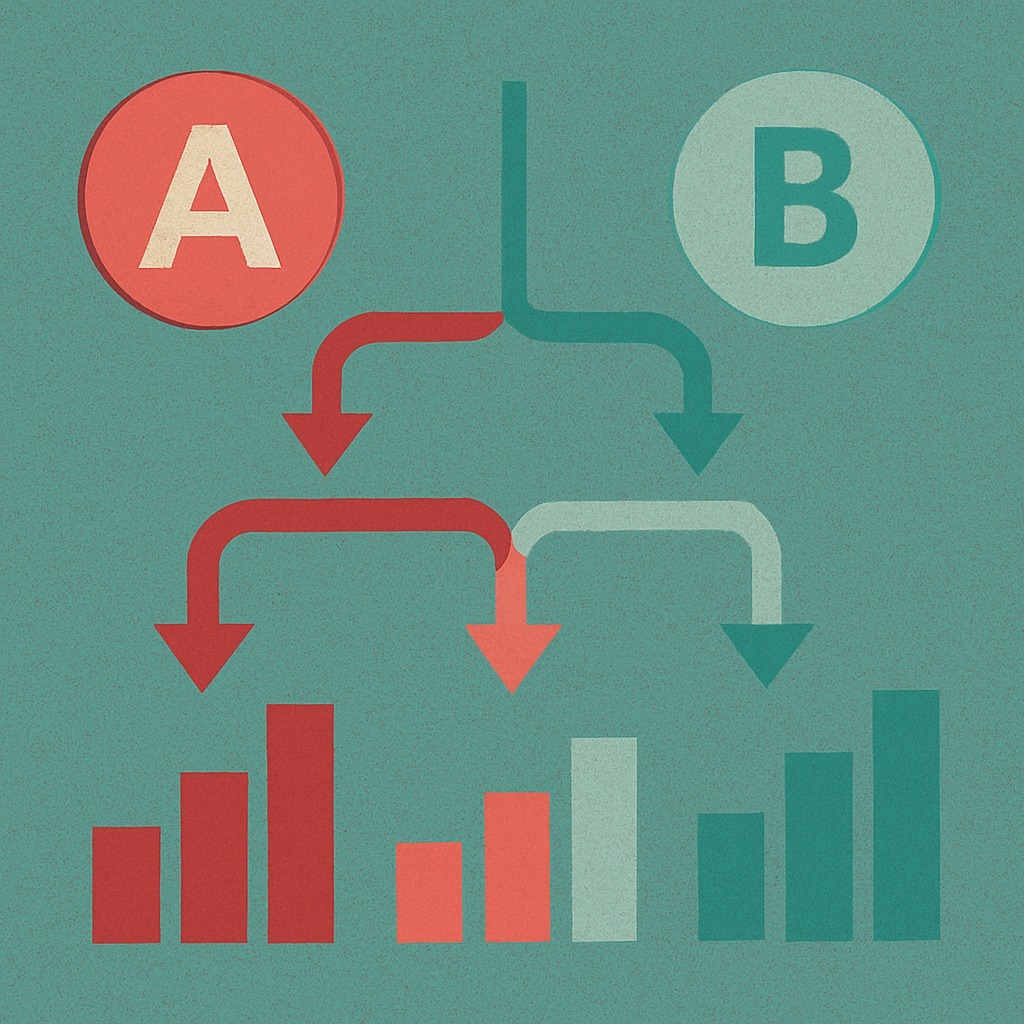
A/B Testing Pitfalls: How Small Mistakes Can Lead to Big Missteps

A/B testing is a powerful tool — but even small mistakes can quietly sabotage your results, sending you down the wrong path with false confidence.
The good news? Most A/B testing mistakes are easy to fix once you know what to watch for.
Here’s a practical guide to avoiding the most common pitfalls so your tests stay strong, clean, and reliable.
1. Peeking at the Results Too Early
The Mistake:
You check your test results a few hours (or days) after launch, see a big swing, and decide to end the test early.
Why It's a Problem:
Early results are unstable. Natural randomness can make one variation look like a big winner — until more data levels it out.
How to Fix It:
-
Set a minimum runtime (at least one full business cycle, ideally 1–2 weeks).
-
Don’t look at test results until you hit your pre-decided sample size or time frame.
-
Commit in advance to the rules for calling a winner.
2. Using Uneven Sample Sizes
The Mistake:
One version accidentally gets way more traffic than the other — or the audience isn’t split truly randomly.
Why It's a Problem:
Biased traffic makes your results unreliable. You’re no longer comparing apples to apples.
How to Fix It:
-
Use testing tools that randomize and evenly split traffic by default (e.g., Google Optimize, Optimizely, VWO).
-
Double-check that variations are getting equal exposure throughout the test period.
3. Testing Too Many Changes at Once
The Mistake:
You change five things in one test — the headline, image, CTA, layout, and offer.
Why It's a Problem:
If one version wins, you won’t know which change made the difference.
How to Fix It:
-
Focus on testing one major change at a time (A/B test).
-
Use multivariate testing only when you have very high traffic and a strong plan to interpret multiple changes.
4. Not Defining a Clear Success Metric
The Mistake:
You launch a test without a clear idea of what "winning" means. You start chasing whatever number looks best after the fact.
Why It's a Problem:
Shifting goals mid-test leads to cherry-picking — and bad decision-making.
How to Fix It:
-
Define your hypothesis and primary success metric before launching.
-
Stick to that metric when analyzing results.
5. Ignoring Statistical Significance
The Mistake:
You declare a winner based on a 5% lift with tiny sample sizes — without checking if it’s statistically significant.
Why It's a Problem:
Without significance, there’s a high chance your "winner" is just random luck.
How to Fix It:
-
Use a calculator to ensure at least 95% confidence in your results.
-
Don't call a test until it reaches the required traffic or event thresholds (often 1,000+ visitors or 100+ conversions per variation).
6. Running Tests for Too Long
The Mistake:
You run a test for weeks or months hoping for a better result.
Why It's a Problem:
User behavior can shift over long periods (seasons, promotions, competitors) making the test invalid. Plus, fatigue can set in with repeat audiences.
How to Fix It:
-
Plan to run tests for 2–4 weeks max unless you have a special reason to extend.
-
Focus on reaching sample size goals, not stretching endlessly.
Bottom Line:
A/B testing works best when you combine curiosity with discipline.
By avoiding these common mistakes, you protect your tests from false wins, wasted time, and misleading insights — and you get smarter, stronger growth strategies as a result.
Want to make your A/B testing process even more reliable? [Contact us] — we’ll help you set up smarter experiments that drive real results.