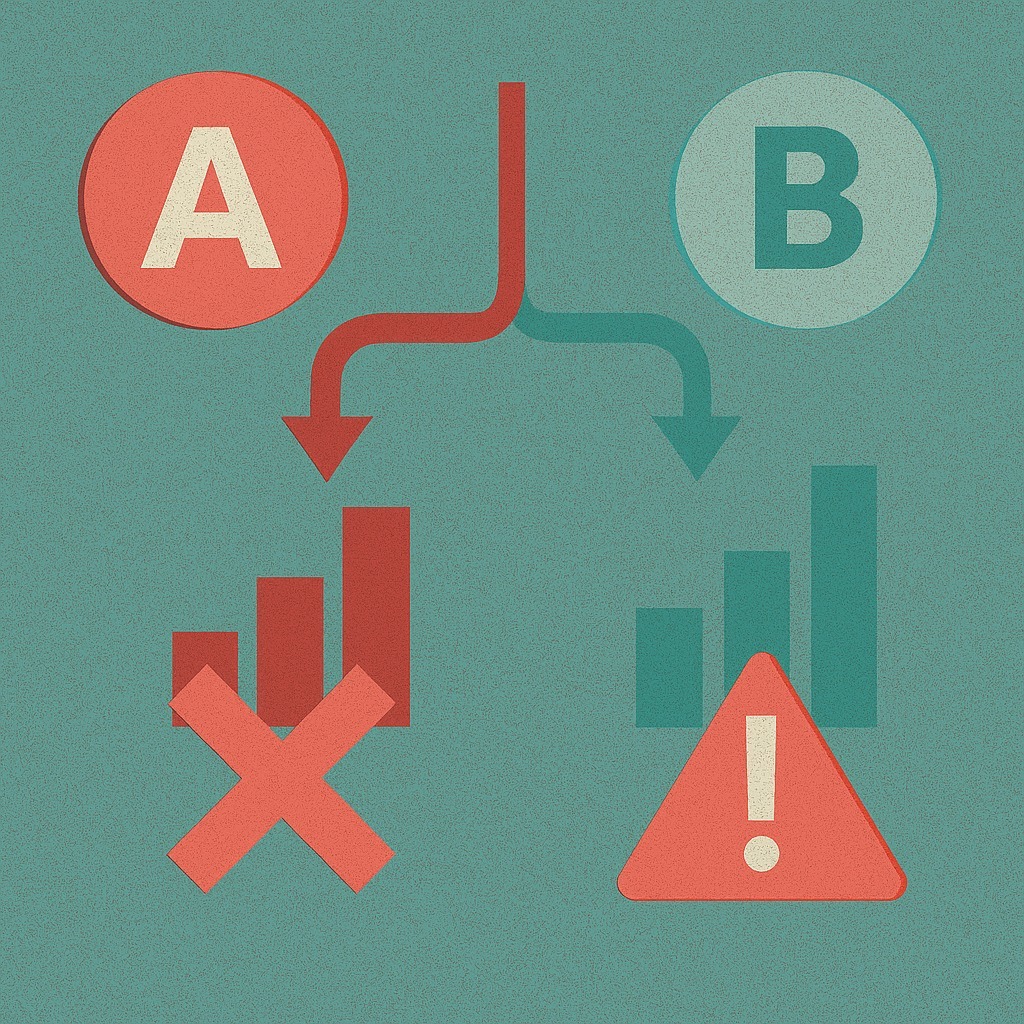
The Hidden Risk of Early Wins: Why Statistical Significance Matters

In the late 19th century, a curious scientist named Francis Galton set out to solve one of agriculture’s biggest problems: how to breed crops that were consistently stronger, healthier, and more productive. Early measurements in his experiments looked promising. Some generations showed dramatic improvements — bigger plants, better yields.
Excited by the early results, Galton and others thought they might have found the secret to reliably improving crops. But as more generations were studied, a pattern emerged: the extreme gains faded back toward the average. This discovery — now known as regression to the mean — became one of the cornerstones of modern statistics.
Galton’s work was a breakthrough not because he "found a super-crop," but because he realized the danger of being fooled by small, early results. His insight laid the groundwork for much of today’s thinking about probability, data analysis, and scientific testing — earning him the title "father of statistics."
If Galton had simply trusted those early numbers, it could have led to wasted resources, failed crops, and dashed hopes — at a time when food security and efficient farming were becoming urgent challenges. Instead, by demanding better evidence, he helped set a higher standard: don’t make decisions on noise.
So what is statistical significance, really?
It’s a way of measuring whether the difference you see between two versions is likely to be real — or just due to random chance. Reaching statistical significance (usually at a 95% confidence level) means you can be reasonably sure your results aren’t a fluke.
Without it, you risk:
-
Rolling out changes that don't actually work
-
Missing out on better-performing ideas
-
Wasting time and resources on "false positives"
How much data do you need?
It depends on your starting conversion rate and how big of a lift you're hoping to detect. (See When Should I A/B Test on My Website? for benchmarks.)
In short:
-
Lower conversion rates = More visitors needed
-
Bigger changes = Easier to detect quickly
-
Small tweaks = Require even more data to prove
If you don't have the traffic yet:
Focus on qualitative insights: user testing, heatmaps, surveys, and strong UX principles. These will get you bigger wins, faster, than running tests on tiny numbers.
If you don't know your baseline metrics:
That's step one. Clean analytics setup is critical. Contact us here — we can help you build a foundation that supports real, confident decision-making.
Bottom line:
Statistical significance protects you from acting on noise instead of insights. In growth marketing, patience isn’t just a virtue — it’s a competitive advantage.